Dagstuhl-Seminar 23361
Multiobjective Optimization on a Budget
( 03. Sep – 08. Sep, 2023 )
Permalink
Organisatoren
- Richard Allmendinger (University of Manchester, GB)
- Carlos M. Fonseca (University of Coimbra, PT)
- Serpil Sayin (Koc University - Istanbul, TR)
- Margaret M. Wiecek (Clemson University, US)
Kontakt
- Marsha Kleinbauer (für wissenschaftliche Fragen)
- Jutka Gasiorowski (für administrative Fragen)
Programm
Multiobjective optimization (MO), a discipline within systems science that provides models, theories, and methodologies to address decision-making problems under conflicting objectives, has a myriad of applications in all areas of human activity ranging from business and management to engineering. This seminar is a result of the desire to continue to make MO useful to society as it faces complex decision-making problems and experiences limited resources for decision making. Of particular interest are processes that evolve competitively in environments with scarce resources and lead to decision problems that are characterized by multiple, incommensurate, and conflicting objectives, and engage multiple decision-makers. Viewing optimization and decision making as the complementary aspects of the multiobjective paradigm, the seminar set out to focus around three major types of resource limitations: methodological (e.g., number of solution evaluations), technical (e.g., computation time, energy consumption), and human related (e.g., decision maker availability and responsiveness). The effect of these limitations on optimization and decision-making quality, as well as methods to quantify and mitigate this influence, were of particular interest. Ideas related to modelling, theory, algorithm design, benchmarking, performance metrics, and novel applications of MO under budget constraints were discussed.
To initiate a discussion among the participants on how to address challenges of MO under a budget, the organizers presented specific research directions at the beginning of the seminar. These directions along with their highlights are described below.
- Model reduction: In the MO problem not all functions may be of interest to the decision maker (DM) or not all objectives may be in conflict with each other. Under a limited budget, it is of interest to make the original problem simpler by removing unnecessary objective functions while the solution set remains unchanged. Another reason to reduce the problem is its size. MO problems with four or more criteria bring computational and decision-making challenges that are not typical when the number of objectives is lower.
- Model decomposition and coordination-based decision making: If a reduction of the objectives is not possible, then the solution of the overall MO problem in its entirety may be challenging or even impossible to obtain. In this situation, decomposition of the MO problem into a set of MO subproblems with a smaller number of criteria becomes appealing provided solving the subproblems can be coordinated and related to solving the original problem. When the MO problem is decomposed while computation of the overall solution set is possible, the decomposition goal is to enhance capability of making coordinated tradeoff decisions by working in lower dimensional spaces, which decreases the cognitive burden on DMs. Otherwise, if computation of the overall solution set is not possible, the decomposition goal becomes more challenging since the intention is to coordinate the subproblems' solution sets to construct the overall set and to facilitate decision making in a similar way.
- Representation of the optimization solution set: It is of interest to design cost-effective methods for obtaining a complete or partial description of the Pareto set. An exact description of this set might be available analytically as a closed-form formula, numerically as a set of points, or in mixed form as a parametrized set of points. Unfortunately, for the majority of MO problems, it is not easy to obtain an exact description of the solution set that includes typically a very large number or infinite number of points. Even if it is theoretically possible to find these points exactly, this is often computationally challenging and expensive, and therefore is usually abandoned. On the other hand, if it is possible to obtain the complete solution set, one might not be interested in this task due to overflow of information. Another reason for approximating the solution set, rather than finding the solution set exactly, is that many real-world problems (e.g., in engineering) cannot be completely and correctly formulated before a solution procedure starts. Since the exact solution set is very often not attainable, an approximated description of the solution set becomes an appealing alternative.
- Surrogate-assisted optimization: The combination of evolutionary MO (EMO) algorithms with efficient computational models, often known as metamodels or surrogates, has become a common approach to approximate outcomes of a time-consuming, expensive, and/or resource intense simulation or physical experiment, and thus to tackle problems with a limited budget. Surrogate-assisted (SA) methods vary in aspects such as the use of the metamodel (e.g., different models for different objective functions or one model for all objective functions), type of metamodel (e.g., Gaussian process, radial basis neural network, etc.), how the metamodel is updated (e.g., expected improvement, expected hypervolume improvement), and training time of the metamodel. In particular, the combination of optimization with Gaussian process approximation, known as Bayesian optimization, is a recent trend to efficiently deploy data in model development.
- Multistage optimization: In real-world applications, problem data does not always become available all at once, but at different points in time until a final decision needs to be made. In particular, waiting until all the required data is available may not leave enough time to run the optimization process on the whole problem and successfully compute a final decision. In addition, it is often possible to model the uncertainty associated with the yet unknown data given the data that is already known, at least to some extent. Two-stage (and, more generally, multi-stage) approaches to optimization reformulate the original problem as a number of sub-problems to be solved sequentially, in such a way that the last problem(s) in the sequence can effectively be solved in the (short) time available.
- Preference acquisition and communication with the decision maker: The ultimate goal in MO is to serve one or multiple DMs whose goal is to come up with a single most preferred solution from among the ones that are available. Given an optimization model, DM's preferences may be incorporated prior to, during or after employing a solution procedure. In particular, interactive methods require the DM's involvement in the solution process during which they reveal their preferences based on the presented information. Under a limited budget, communication with the DM shall be designed effectively and economically.
- Benchmarking of algorithms: SA methods are considered as the method of choice to tackle problems subject to a limited budget in terms of function evaluations. However, SA methods are not often compared to widely different alternatives (e.g., different kernels and distance measures, non-SA methods, etc.), and are often tested on narrow sets of problems (multimodal, low-dimensional, static, deterministic, unconstrained, and continuous functions) and rarely on real-world problems, which makes it difficult to assess where (or if) these methods actually achieve state-of-the-art performance in practice. Moreover, several aspects in the design of SA algorithms vary across implementations without a clear recommendation emerging from current practices, and many of these design choices are not backed up by authoritative test campaigns. This seminar topic aimed to raise awareness and hence a push to more work being carried out on developing benchmarking guidelines for SA algorithms.
In response to the presented research directions, some participants found research topics of interest among those suggested by the organizers. These topics included model reduction, decomposition and coordination, solution set representation, and surrogate modeling. Other participants proposed different topics that also targeted the theme of MO under a budget. Those topics included design of experiments for MO, correlations in MO, and design of evolutionary algorithms. Overall, seven research topics were proposed and pursued.
Independently of developing and forming research topics, a collection of eight talks were given during the seminar. Two of the speakers were considered "invited" because they were asked before the seminar to give a talk. These talks addressed two of the research directions initiated by the organizers. The other speakers, being inspired by the ongoing seminar, proposed talks that were integrated daily into the seminar program. The invited and contributed talks kept the seminar in balance ensuring ample time for working in groups.
During the seminar the schedule was updated on a daily basis to maintain flexibility in balancing time slots for the invited and contributed talks, discussions, and working group sessions. The working groups were established on the first day in an interactive fashion. Starting with three large working groups focused around the three central topics of the seminar (methodological, technical, and human-related resource limitations), participants were invited to formulate their favorite topics and most important challenges. The three initial groups split to eventually form eight groups by the end of the seminar. During the week the participants were allowed to change the working groups based on their research interest. The abstracts of the delivered talks and the extended abstracts of the working groups can be found in the subsequent chapters of the full report.
Further notable events during the week included: (i) a hike that took place on Wednesday afternoon, (ii) a session allowing the participants to share the details of upcoming professional events in the research community, (iii) a joint session with the participants of the concurrent seminar 23362 ``Decision-Making Techniques for Smart Semiconductor Manufacturing'' and (iv) an informal get together on Thursday evening.
Offers and Needs Market
An Offers & Needs Market ran throughout the entire week. The participants could write their research offers and needs regarding MO on note paper in different colors and post them on pin boards (see Fig. 1) to attract or find a possible collaborator. Participants discussed potential collaboration opportunities during the coffee breaks and after hours.
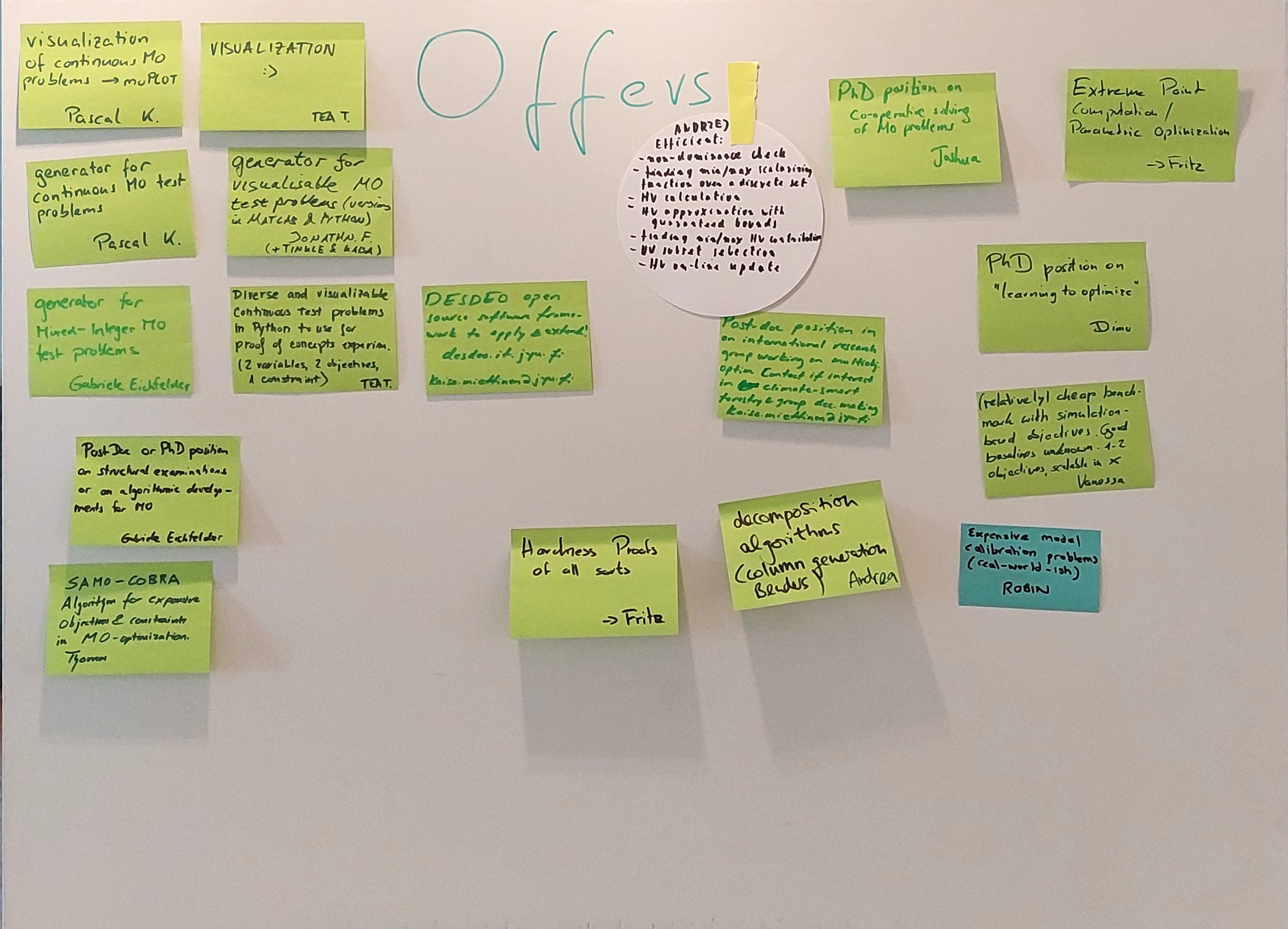
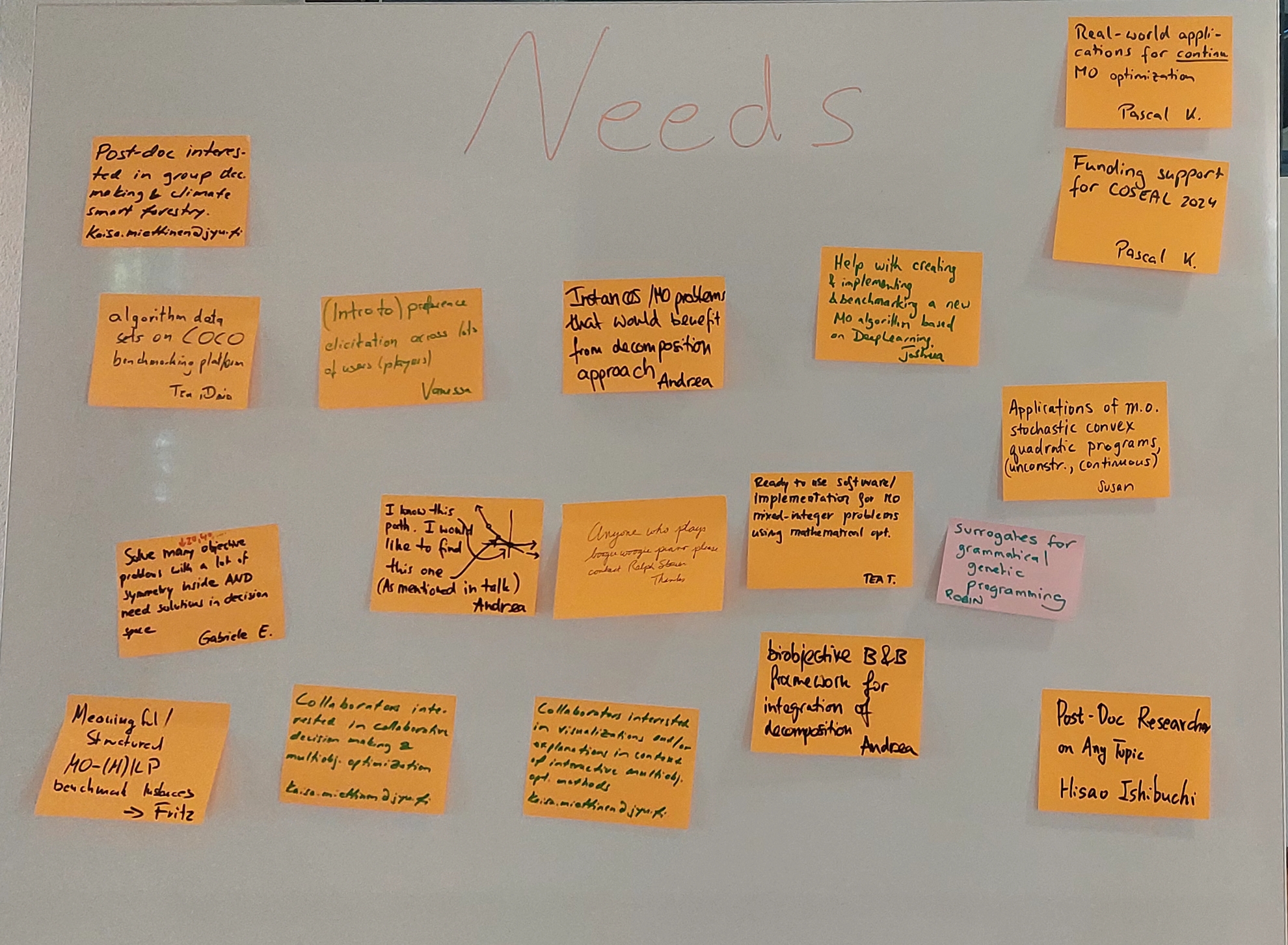
Outcomes
The outcomes of each of the working groups can be seen in the sequel.
The organizers have arranged a special issue of the Journal of Multi-Criteria Decision Analysis entitled "Multiobjective Optimization on a Budget" for which they will serve as Guest Editors. This issue will be an outlet for papers authored and submitted by the seminar's participants as well as by researchers world-wide.
This seminar resulted in a very insightful, productive and enjoyable week. It has already led to first new results, cooperations and research topics.
Acknowledgements
The organizers would like to express their appreciation to the Dagstuhl Office and its helpful and patient staff for their professional support and smooth cooperation; huge thanks to the organizers of the previous seminars in this series for setting us up for success; and thanks to all the participants, who worked hard and were amiable company all week.
In the full report, we also give special thanks to Margaret Wiecek as she steps down from the organizer role.

Multiobjective optimization (MO), a discipline within systems science that provides models, theories, and methodologies to address decision-making problems under conflicting objectives, has a myriad of applications in all areas of human activity, ranging from business and management to engineering. The proposed seminar is motivated by the desire to continue to make MO useful to society as it faces complex decision-making problems and experiences limited resources for decision making. Of particular interest are processes that evolve competitively in environments with scarce resources and lead to decision problems that are characterized by multiple, incommensurate, and conflicting objectives, and engage multiple decision-makers.
The Dagstuhl Seminar will focus on three major types of resource limitations: methodological (e.g., number of solution evaluations), technical (e.g., computation time, energy consumption), and human-related (e.g., decision maker availability and responsiveness). The effect of these limitations on optimization and decision-making quality, as well as methods to quantify and mitigate this influence, will be of particular interest. These methods include reduction and decomposition of optimization and decision-making models; representation of solution sets; different types of optimization approaches such as coordination-based, Bayesian, and multi-stage; preference acquisition; and benchmarking of algorithms. Applications within engineering-design optimization, simulation optimization, and experiment-based optimization will serve as motivation.
Following on the tradition of earlier Dagstuhl Seminars on multiobjective optimization, the seminar will be a platform for experts in two main research communities - Evolutionary MO (EMO) and Multiobjective Decision Making (MCDM) - to propose and discuss novel ideas related to modeling, theory, and applications of MO under competitive conditions and limited budgets.

- Thomas Bäck (Leiden University, NL) [dblp]
- Mickaël Binois (INRIA - Sophia Antipolis, FR) [dblp]
- Fritz Bökler (Universität Osnabrück, DE) [dblp]
- Jürgen Branke (University of Warwick, GB) [dblp]
- Dimo Brockhoff (INRIA Saclay - Palaiseau, FR) [dblp]
- Tinkle Chugh (University of Exeter, GB) [dblp]
- Kerstin Dächert (HTW Dresden, DE) [dblp]
- Benjamin Doerr (Ecole Polytechnique - Palaiseau, FR) [dblp]
- Matthias Ehrgott (Lancaster University, GB) [dblp]
- Gabriele Eichfelder (TU Ilmenau, DE) [dblp]
- Jonathan Fieldsend (University of Exeter, GB) [dblp]
- Carlos M. Fonseca (University of Coimbra, PT) [dblp]
- Susan R. Hunter (Purdue University, US) [dblp]
- Ekhine Irurozki (Telecom Paris, FR) [dblp]
- Hisao Ishibuchi (Southern Univ. of Science and Technology - Shenzen, CN) [dblp]
- Andrzej Jaszkiewicz (Poznan University of Technology, PL) [dblp]
- Pascal Kerschke (TU Dresden, DE) [dblp]
- Kathrin Klamroth (Universität Wuppertal, DE) [dblp]
- Joshua D. Knowles (Schlumberger Cambridge Research, GB) [dblp]
- Karl Heinz Küfer (Fraunhofer ITWM - Kaiserslautern, DE) [dblp]
- Arnaud Liefooghe (University of Lille, FR) [dblp]
- Kaisa Miettinen (University of Jyväskylä, FI) [dblp]
- Juliane Mueller (NREL - Golden, US) [dblp]
- Boris Naujoks (TH Köln, DE) [dblp]
- Aneta Neumann (University of Adelaide, AU) [dblp]
- Frank Neumann (University of Adelaide, AU) [dblp]
- Markus Olhofer (HONDA Research Institute Europe GmbH - Offenbach, DE) [dblp]
- Luís Paquete (University of Coimbra, PT) [dblp]
- Robin Purshouse (University of Sheffield, GB) [dblp]
- Alma Rahat (Swansea University, GB) [dblp]
- Andrea Raith (University of Auckland, NZ) [dblp]
- Enrico Rigoni (ESTECO SpA - Trieste, IT) [dblp]
- Stefan Ruzika (RPTU - Kaiserslautern, DE) [dblp]
- Serpil Sayin (Koc University - Istanbul, TR) [dblp]
- Anita Schöbel (Fraunhofer ITWM - Kaiserslautern, DE) [dblp]
- Britta Schulze (Universität Wuppertal, DE) [dblp]
- Ralph E. Steuer (University of Georgia, US) [dblp]
- Michael Stiglmayr (Universität Wuppertal, DE) [dblp]
- Tea Tusar (Jozef Stefan Institute - Ljubljana, SI) [dblp]
- Daniel Vanderpooten (University Paris-Dauphine, FR) [dblp]
- Vanessa Volz (modl.ai - Copenhagen, DK) [dblp]
- Hao Wang (Leiden University, NL) [dblp]
- Margaret M. Wiecek (Clemson University, US) [dblp]
- Kaifeng Yang (Univ. of Applied Sciences - Hagenberg, AT)
Verwandte Seminare
- Dagstuhl-Seminar 04461: Practical Approaches to Multi-Objective Optimization (2004-11-07 - 2004-11-12) (Details)
- Dagstuhl-Seminar 06501: Practical Approaches to Multi-Objective Optimization (2006-12-10 - 2006-12-15) (Details)
- Dagstuhl-Seminar 09041: Hybrid and Robust Approaches to Multiobjective Optimization (2009-01-18 - 2009-01-23) (Details)
- Dagstuhl-Seminar 12041: Learning in Multiobjective Optimization (2012-01-22 - 2012-01-27) (Details)
- Dagstuhl-Seminar 15031: Understanding Complexity in Multiobjective Optimization (2015-01-11 - 2015-01-16) (Details)
- Dagstuhl-Seminar 18031: Personalized Multiobjective Optimization: An Analytics Perspective (2018-01-14 - 2018-01-19) (Details)
- Dagstuhl-Seminar 20031: Scalability in Multiobjective Optimization (2020-01-12 - 2020-01-17) (Details)
- Dagstuhl-Seminar 26041: Uncertainty Quantification in Multiobjective Optimization (2026-01-18 - 2026-01-23) (Details)
Klassifikation
- Machine Learning
- Neural and Evolutionary Computing
- Systems and Control
Schlagworte
- decision making
- expensive optimization
- few-shot learning
- evolutionary algorithms
- simulation optimization